Working AI: Transforming Real Estate With Jasjeet Thind
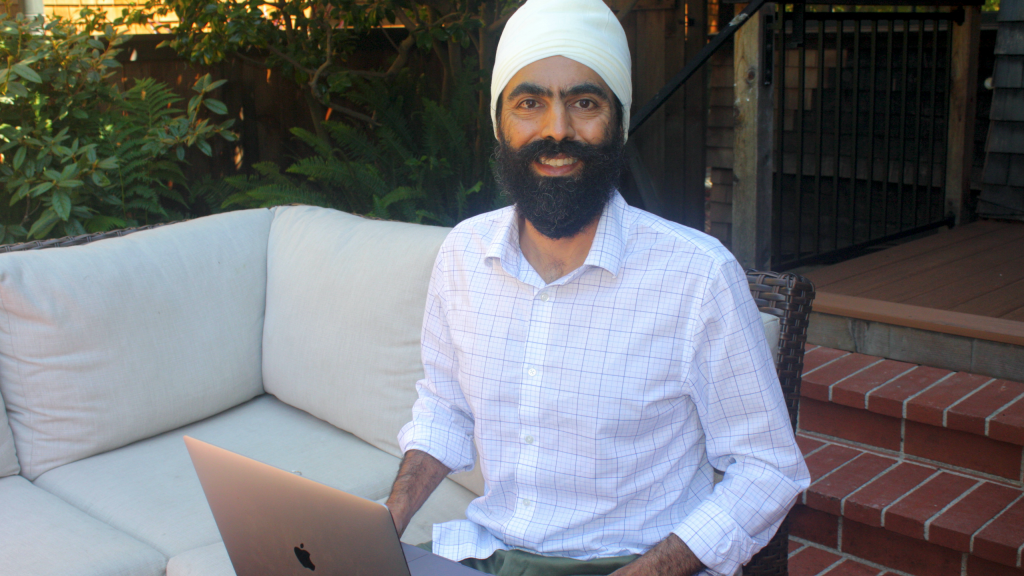
Title: Vice President of Artificial Intelligence at Zillow
Location: Seattle, WA
Education: Cornell University, MS and BS in Computer Science with a specialization in data mining
Favorite ML area: Any area that applies AI to solve real customer problems
Favorite ML researcher: The open source community at large for decades of work developing and scaling machine learning algorithms
As a child, Jasjeet Thind led the other kids at his daycare to engage in shenanigans. He still exercises his leadership skills, but instead he uses them to organize Zillow’s army of engineers as they aim to automate everything about real estate. Thind became fascinated with data as a college student at Cornell University in the 1990s, and his career exploded along with the deep learning revolution. After early jobs writing code, he transitioned into management at Microsoft and then Yahoo, where he oversaw the company’s pioneering recommendation programs. Jasjeet shared his memories of the industry’s early days, how he is building an all-in-one pipeline for home sales, and why new engineers need to balance academic achievement with practical experience.
In high school and middle school, I was always very interested in software development. My first job in high school was as a programmer for a graphics company. In college I got introduced to data mining, and it became my specialization.
You attended Cornell University in the 1990s, before many people were talking about the value of data. How did you become interested in data mining?
You’re right, not many people talked about AI back then. But the classes I enjoyed the most were around algorithms and data mining. It was a lot about pattern matching. But it wasn’t really until I was at Yahoo, doing machine learning for content personalization, that I got to do it at scale.
Yahoo was one of earlier pioneers of this, with personalized news, sports, and other content for a billion-plus monthly users. Very few companies were doing that at the time. It was quite novel to personalize 50,000 to 100,000 content items per user, for a billion-plus monthly users. That was really exciting. Now it’s not uncommon to scale that out to a billion content items per user.
You moved into management at Microsoft just a few years out of college. Why did you make that shift, and what’s it like to manage large-scale engineering projects?
It was a big decision for me. I decided to go for it because I’ve always enjoyed leadership roles. I had some leadership at clubs at university, and when I was young, I would take a leadership role among the other kids at childcare and convince them to do mischievous things. I was the kid leading other kids into trouble a lot. They looked to me to lead the way at the expense of direction from the adults. I’d often persuade all the other kids to go play outside after the adults told us, multiple times, to stay inside and read our books. [Laughs]
You came to Zillow five years ago. What kind of machine learning were they doing when you joined?
So, Zillow started about fifteen years ago, and they are at core a data and analytics company. One of the first products Zillow launched was Zestimate, which is an analytics tool for home pricing. Back in 2006, Zillow had Zestimates on around 40 million homes. The company realized that its users were essentially looking at the listed prices of nearby homes to figure out the price on the house they were thinking of buying. To automate that for 40 million homes, you have to leverage an analytical solution. We’re still investing in Zestimate and it’s now used to estimate the prices of 100 million-plus homes. And, we’re leveraging more sophisticated algorithms, like analyzing images and listing descriptions using deep learning.
Tell me more about how you are using computer vision and NLP with Zestimate.
The images a seller posts with their Zillow listing often have attributes that provide information that is not in the listing. For example, whether a house has high-end stainless steel appliances or marble countertops. We automatically extract these features with deep learning and process them as inputs to help determine the price of the home.
And we’re inventing a natural language search. It’s cumbersome to find the right home, so our natural language search allows people to type or say in words what they are looking for.
Are you using machine learning for areas outside of Zestimate?
When I joined, Zillow was primarily helping people find a home. Now we’re making it easier to buy one. We’re investing in areas such as Natural Language Search, 3D tours, conversational AI, document processing and understanding. We’re expanding our charter to streamline the entire transaction of buying, selling, or renting.
For instance, when you are in the process of buying a home, you have to get a pre-approval letter from your lender, go through title insurance, and read through a thick stack of other documents. If you are renting, you have an application and lease. There’s so much documentation and red tape, it’s cumbersome. We’re working on a conversational natural language processing system with document understanding to enable two-way conversations that will streamline these processes.
We’ve also invested in computer vision and deep learning to automatically generate 3D tours, so prospective buyers can get an immersive experience. People moving to different cities don’t have to get on an airplane to tour homes they are interested in. Also, with the Covid situation, it’s hard to go inside a home, so now people can see homes from wherever they are.
What is your dream project?
I’m a huge real estate geek, so my dream would be to have a robotic offer on every home. With Zillow Offers we are buying and selling homes, and it’s the very start of automating the entire process. We want to get to the point where buying homes is similar to buying products on ecommerce.
How do you continue learning?
Learning is an everyday thing, and you have to keep doing it, otherwise you’ll fall behind. At work, I learn by having the opportunity to work on hard problems. I learn from listening to my teams and bouncing ideas off of machine learning engineers, data scientists, and applied scientists. I read a lot of online blogs, our own material. That’s all on the AI side. On the management side, I’m always learning to be better as well.
What are your thoughts on diversity in the machine learning industry? What can companies be doing to encourage diversity, and what are you doing as a manager?
Increasing diversity in AI and the tech industry at-large is still a huge, persistent problem. We’re taking intentional steps to diversify our own workforce, like leveraging algorithms to analyze our job postings and create inclusive job descriptions with gender-neutral language, significantly expanding our recruitment program to engage with more historically Black colleges and universities, and instituting benchmarks to ensure higher representation of qualified diverse candidates in our hiring pipeline. We also just introduced a new flexible work-from-home policy, since Covid has all of us working from home. We’re still working out the details, but this new policy will definitely provide more opportunities to hire diverse and talented tech workers living outside our big office hubs like Seattle, San Francisco, and New York City.
I get really excited for the AI Forum event we host every year. We bring together top industry and academic AI practitioners with grad students and people early in their career to talk about their latest research and what it’s like working in industry. It’s a great way to meet brilliant, diverse people who want to break into AI. This year the forum was virtual, so we were able to reach people from anywhere.
What advice do you have for people hoping to break into AI?
Take advantage of all the resources there are online that combine theoretical concepts with practical experience. Deeplearning.ai is a great example of that. I also think people should work hard to get a good internship. Many companies are hiring interns. Do anything that gives you actual experience to help solidify the concepts you learn.
What problems do you think AI is well-positioned to solve?
I could think of a few, but one that comes to mind is health care. It’s been a challenge to advance AI so that it helps diagnose patients, come up with other health solutions, while also protecting patient privacy. That’s a legit challenge